In the most of the works for implementation and control of parallel robots, especially Generally, in parallel robots, solving the forward kinematic problem is much harder than finding the inverse kinematic solution, thus, workspace determination using FKP is a demanding task in most cases. Therefore, in this research, a progressive growing neural gas network algorithm (PGNGN) is proposed, which is a systematic and general approach to obtain the topology of the workspace based on IKP equations.
After establishing a preliminary network with few initial data points, the network starts to develop itself by considering new data points around its border neurons through a boundary data generation procedure. The proposed algorithm is able to continue learning, adding units and connections, until a performance criterion has been met which leads to a clear workspace topology with minimal errors.
The most important features of this algorithm are the abilities to detect cavities in the workspace, consider singularities and distinguish separated regions of the workspace. Till now, workspace of various parallel robots including 3-RRR, 3-RPR and 3-PRR mechanisms and a Gouph-Stewart platform have been determined. Results reveal the applicability and reliability of this method.
Then upon apply different levels of input voltage on the actuator, different step responses are studied in the presence of dead zone, delay and saturation. Through a linear identification process, a nominal second-order linear model is estimated for the actuator. Based on the identified model, the position (length) of the actuator is controlled by applying some straightforward and reliable control solutions.
|
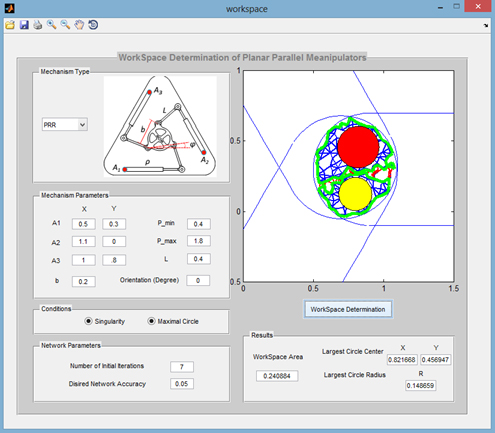 |